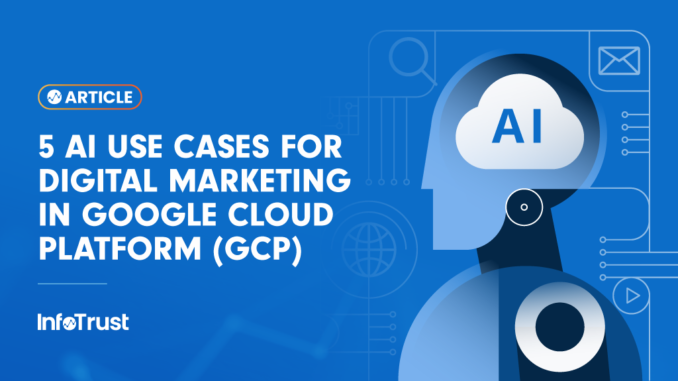
Artificial intelligence (AI) has become a hot topic in recent years, and for good reason. AI has the potential to revolutionize many industries, including digital marketing. Although there’s ample hype and promise surrounding AI, many marketing teams struggle with knowing where to start. In this article, we’ll discuss five AI-powered marketing use cases that your team can implement in Google Cloud Platform (GCP) to drive real results.
AI-powered audiences
Audiences continue to be a key area of focus for marketers and there are a variety of AI and machine learning models in GCP that can be used for :
The type of machine learning model that is best for building a marketing audience will depend on the specific goals of the marketing campaign. For example, if the goal of the campaign is to increase sales, then a classification model could be used to predict which customers are likely to purchase a product. If the goal of the campaign is to increase brand awareness, then a clustering model could be used to group customers together based on their interests.
Whether it’s using the pre-built models or custom models in or , leveraging the power of AI for audience building can lead to improved accuracy, lower costs, and better results.
Personalized marketing messaging
Large language models (LLMs) can be used to write personalized marketing messaging by generating text that is tailored to the specific interests and needs of each individual customer. To do this, the marketing team would supply the context for the message and applicable customer data and the LLM would then use this information to generate text that is relevant and engaging to the customer.
Within GCP, marketers can work within in GCP to leverage Google’s pre-built language models as-is or they can fine-tune the prompts or the models themselves for their use case. For example, marketers could update the model to speak in their brand’s specific voice by supplying additional data for the model to ingest.
There are several benefits to using LLMs to write personalized marketing content. First, it can help to improve the effectiveness of marketing campaigns. When customers receive marketing content that is relevant to their interests, they are it and take action. Second, it can help to build relationships with customers. Customers now from brands and consumers reward companies that excel at personalization with greater loyalty. Third, it can help shorten the time needed to launch campaigns and reduce costs. By automating the process of writing marketing content, brands can strengthen their relationships with customers while saving time and money that can be used for other purposes.
AI-powered creative analysis
AI models can be used to analyze digital marketing campaign assets, such as , , and , to identify patterns and trends that can be used to improve the effectiveness of future campaigns. For example, AI models can be used to identify which images are most likely to be clicked on, which videos are most likely to be watched, and which text is most likely to be read. This information can then be used to select the most effective assets for future campaigns. By using creative analysis in this way, marketers can improve campaign effectiveness, reduce costs, and shorten the time needed to launch new campaigns.
Another benefit of using AI for improving marketing assets is that this form of campaign optimization does not require personal data. Marketers are under pressure to adapt to the industry-wide changes in the privacy landscape that are making it harder to reach new customers and retarget prospects. AI-powered creative analysis is a privacy-centric way to use first-party data to drive better campaign performance.
Product and search recommendations
Marketers can harness the power of Google’s pre-built AI models via for Retail, a suite of machine learning services in GCP, to improve the customer experience and increase sales. Discovery AI for Retail includes a variety of features, such as:
The Discovery AI utilizes product catalog and user interaction data for training the models. Plus, since it’s a machine learning service, it’s also scalable and can be used to support large retailers with a high volume of traffic. By using Discovery AI, marketers can quickly implement and test these pre-built models on their own digital properties and ultimately produce a better experience for customers and higher revenue for the brand.
Forecasting models can be used in digital marketing to which can then be used to improve the effectiveness of marketing campaigns. One common use case is to build models that predict the generated by different channels and tactics. The insights from these types of models are used for allocating marketing budgets more effectively and setting realistic sales targets.
In addition to prediction, forecasting models can also be used to identify leading indicators of conversions and other KPIs. Being able to recognize these leading indicators gives marketers even greater visibility into what may happen next which enables them to better plan for the future and improve decision making.
Leveraging AI technology for digital marketing has never been easier. However, in order to do so, it’s imperative that your brand has a solid analytics foundation. This includes having a and architecture for collecting first-party data, to ensure that the data is clean and of high quality, and making it accessible in the cloud to your end users. With these prerequisites in place, your team is well positioned to drive greater efficiency and better results using AI.
Leave a Reply