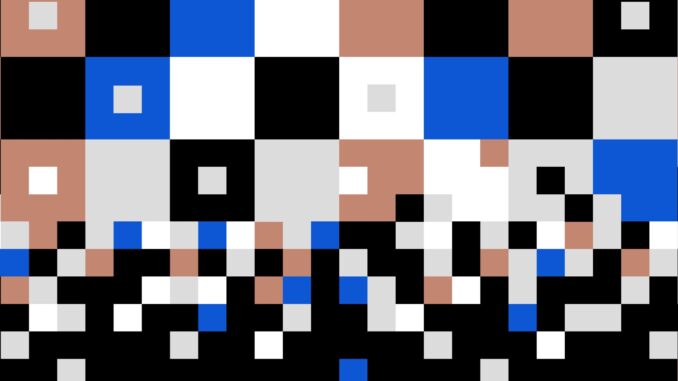
Alphabet’s DeepMind lost $572 million last year. What does it mean?
DeepMind, likely the world’s largest research-focused artificial intelligence operation, is losing a lot of money fast, more than $1 billion in the past three years. DeepMind also has more than $1 billion in debt due in the next 12 months.
Does this mean that AI is falling apart?
Gary Marcus is founder and CEO of Robust.AI and a professor of psychology and neural science at NYU. He is the author, with Ernest Davis, of the forthcoming Rebooting AI: Building Artificial Intelligence We Can Trust.
Not at all. Research costs money, and DeepMind is doing more research every year. The dollars involved are large, perhaps more than in any previous AI research operation, but far from unprecedented when compared with the sums spent in some of science’s largest projects. The Large Hadron Collider costs something like $1 billion per year and the total cost of discovering the Higgs Boson has been estimated at more than $10 billion. Certainly, genuine machine intelligence (also known as artificial general intelligence), of the sort that would power a Star Trek–like computer, capable of analyzing all sorts of queries posed in ordinary English, would be worth far more than that.
Still, the rising magnitude of DeepMind’s losses is worth considering: $154 million in 2016, $341 million in 2017, $572 million in 2018. In my view, there are three central questions: Is DeepMind on the right track scientifically? Are investments of this magnitude sound from Alphabet’s perspective? And how will the losses affect AI in general?
On the first question, there is reason for skepticism. DeepMind has been putting most of its eggs in one basket, a technique known as deep reinforcement learning. That technique combines deep learning, primarily used for recognizing patterns, with reinforcement learning, geared around learning based on reward signals, such as a score in a game or victory or defeat in a game like chess.
DeepMind gave the technique its name in 2013, in an exciting paper that showed how a single neural network system could be trained to play different Atari games, such as Breakout and Space Invaders, as well as, or better than, humans. The paper was an engineering tour de force, and presumably a key catalyst in DeepMind’s January 2014 sale to Google. Further advances of the technique have fueled DeepMind’s impressive victories in Go and the computer game .
The trouble is, the technique is very specific to narrow circumstances. In playing Breakout, for example, tiny changes—like moving the paddle up a few pixels—can cause dramatic drops in performance. DeepMind’s StarCraft outcomes were similarly limited, with better-than-human results when played on a single map with a single “race” of character, but poorer results on different maps and with different characters. To switch characters, you need to retrain the system from scratch.
LEARN MORE
The WIRED Guide to Artificial Intelligence
In some ways, deep reinforcement learning is a kind of turbocharged memorization; systems that use it are capable of awesome feats, but they have only a shallow understanding of what they are doing. As a consequence, current systems lack flexibility, and thus are unable to compensate if the world changes, sometimes even in tiny ways. (DeepMind’s recent results with kidney disease have been questioned in similar ways.)
Deep reinforcement learning also requires a huge amount of data—e.g., millions of self-played games of Go. That’s far more than a human would require to become world class at Go, and often difficult or expensive. That brings a requirement for Google-scale computer resources, which means that, in many real-world problems, the computer time alone would be too costly for most users to consider. By one estimate, the training time for AlphaGo cost $35 million; the same estimate likened the amount of energy used to the energy consumed by 12,760 human brains running continuously for three days without sleep.
But that’s just economics. The real issue, as Ernest Davis and I argue in our forthcoming book Rebooting AI, is trust. For now, deep reinforcement learning can only be trusted in environments that are well controlled, with few surprises; that works fine for Go—neither the board nor the rules have changed in 2,000 years—but you wouldn’t want to rely on it in many real-world situations.
Little Commercial Success
In part because few real-world problems are as constrained as the games on which DeepMind has focused, DeepMind has yet to find any large-scale commercial application of deep reinforcement learning. So far Alphabet has invested roughly $2 billion (including the reported $650 million purchase price in 2014). The direct financial return, not counting publicity, has been modest by comparison, about $125 million of revenue last year, some of which came from applying deep reinforcement learning within Alphabet to reduce power costs for cooling Google’s servers.
Deep reinforcement learning could be like the transistor, a research invention that changed the world, or it could be a “solution in search of problem.”
What works for Go may not work for the challenging problems that DeepMind aspires to solve with AI, like cancer and clean energy. IBM learned this the hard way when it tried to take the Watson program that won Jeopardy! and apply it to medical diagnosis, with little success. Watson worked fine on some cases and failed on others, sometimes missing diagnoses like heart attacks that would be obvious to first-year medical students.
Of course, it could simply be an issue of time. DeepMind has been working with deep reinforcement learning at least since 2013, perhaps longer, but scientific advances are rarely turned into product overnight. DeepMind or others may ultimately find a way to produce deeper, more stable results with deep reinforcement learning, perhaps by bringing it together with other techniques—or they may not. Deep reinforcement learning could ultimately prove to be like the transistor, a research invention from a corporate lab that utterly changed the world, or it could be the sort of academic curiosity that John Maynard Smith once described as a “solution in search of problem.” My personal guess is that it will turn out to be somewhere in between, a useful and widespread tool but not a world-changer.
Nobody should count DeepMind out, even if its current strategy turns out to be less fertile that then many have hoped. Deep reinforcement learning may not be the royal road to artificial general intelligence, but DeepMind itself is a formidable operation, tightly run and well funded, with hundreds of PhDs. The publicity generated from successes in Go, Atari, and StarCraft attract ever more talent. If the winds in AI shift, DeepMind may be well placed to tack in a different direction. It’s not obvious that anyone can match it.
Meanwhile, in the larger context of Alphabet, $500 million a year isn’t a huge bet. Alphabet has (wisely) made other bets on AI, such as Google Brain, which itself is growing quickly. Alphabet might change the balance of its AI portfolio in various ways, but in a $100 billion-a-year revenue company that depends on AI for everything from search to advertising recommendation, it’s not crazy for Alphabet to make several significant investments.
Concerns of Overpromising
The last question, of how DeepMind’s economics will affect AI in general, is hard to answer. If hype exceeds delivery, it could bring on an “AI winter,” where even supporters are loath to invest. The investment community notices significant losses; if DeepMind’s losses were to continue to roughly double each year, even Alphabet might eventually feel compelled to pull out. And it’s not just the money. There’s also the lack of tangible financial results thus far. At some point, investors might be forced to recalibrate their enthusiasm for AI.
It’s not just DeepMind. Many advances promised just a few years ago—such as cars that can drive on their own or chatbots that can understand conversations—haven’t yet materialized. Mark Zuckerberg’s April 2018 promises to Congress that AI would soon solve the fake news problem have already been tempered, much as Davis and I predicted. Talk is cheap; the ultimate degree of enthusiasm for AI will depend on what is delivered.
For now, genuine machine intelligence has been easier to hype than to build. While there have been great advances in limited domains like advertising and speech recognition, AI unquestionably still has a long way to go. The benefits from sound analysis of large data sets cannot be denied; even in limited form, AI is already a powerful tool. The corporate world may become less bullish about AI, but it can’t afford to pull out altogether.
My own guess?
Ten years from now we will conclude that deep reinforcement learning was overrated in the late 2010s, and that many other important research avenues were neglected. Every dollar invested in reinforcement learning is a dollar not invested somewhere else, at a time when, for example, insights from the human cognitive sciences might yield valuable clues. Researchers in machine learning now often ask, “How can machines optimize complex problems using massive amounts of data?” We might also ask, “How do children acquire language and come to understand the world, using less power and data than current AI systems do?” If we spent more time, money, and energy on the latter question than the former, we might get to artificial general intelligence a lot sooner.
More Great WIRED Stories
This content was originally published here.
Leave a Reply